Research and Discovery
What mechanisms shape the activity of our genes?
How does gene activity shape brain function, behavior and disease risks?
Discovery of Brain-Enriched Noncanonical Genomic Imprinting.
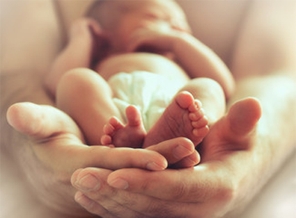
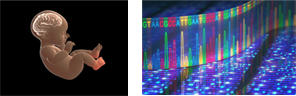
We had an early role in the development and application of next generation sequencing technologies to study allele-specific expression effects using RNA-seq. This was a challenging initiative at the time, since RNASeq protocols had not been published when the project began in early 2007 and analysis methods did not exist, yet. We were one of the first to publish allele-specific RNA-seq, and we used this methodology to perform comparative profiling of genomic imprinting effects in different brain regions, between males and females and at different developmental stages of brain development. The study was the first of its kind and challenged thinking in the field by revealing many genes that exhibit a maternal or paternal allele expression bias. Canonical genomic imprinting involves complete silencing of one parental allele. We now call these maternal and paternal allele expression biases, noncanonical imprinting effects. More recently, my lab developed improved methods for studying noncanonical imprinting, which revealed that it is (i) more prevalent in the mouse genome than canonical imprinting, (ii) is a highly tissue specific form of gene regulation and (iii) is more prevalent in the brain compared to non-neural tissues. We determined that noncanonical imprinting exists in wild populations of mice and, by developing a new RNA in situ hybridization strategy to resolve allelic expression at the cellular level, we discovered that noncanonical imprinting is a highly cell type specific form of imprinting that impacts allelic expression in discrete subpopulations of brain cells. Finally, we discovered that noncanonical imprinting effects can significantly shape offspring behavior.
Our research in this area has uncovered novel forms of genomic imprinting and parental influence on brain gene expression in offspring. Our ongoing work is focused on learning how maternally and paternally expressed noncanonical imprinted genes control offspring brain function and behavior and identifying the brain cell types that are affected for different noncanonical imprinted genes. This work advances our understanding of the gene regulatory mechanisms shaping behavior and disease.
Select Papers:
a. Gregg C, Zhang J, Weissbourd B, Luo S, Schroth GP, Haig D, et al. High-resolution analysis of parent-of-origin allelic expression in the mouse brain. Science. 2010 Aug 6;329(5992):643–8. PMCID: PMC3005244
b. Gregg C, Zhang J, Butler JE, Haig D, Dulac C. Sex-specific parent-of-origin allelic expression in the mouse brain. Science. 2010 Aug 6;329(5992):682–5. PMCID: PMC2997643
c. Bonthuis PJ, Huang WC, Stacher Hörndli CN, Ferris E, Cheng T, Gregg C. Noncanonical genomic imprinting effects in offspring. Cell Reports. 2015 Aug 11; 12(6):979-91. PMID: 26235621 (research article)
d. Gregg C, Ferris E. Methods for Detecting Rapidly Processed Introns to Evaluate Allelic Expression. U.S. Patent No. 62/494,162. (2016) Washington, D.C.:U.S. Patent and Trademark Office.
Discovery of Novel Non-Genetic Allele Expression Effects in the Mouse, Macaque and Human Brain.
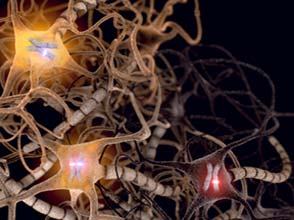
My lab developed innovative genomics and statistical approaches to search for novel forms of gene regulation at the allele level in vivo in the mouse and primate brain. We uncovered genes that differentially express their maternal and paternal alleles and the effects are not due to genomic imprinting or genetic variation. We call this phenomenon differential allele expression (DAE). We uncovered hundreds of genes with DAE in the mouse brain and show that genes with these effects exhibit random monoallelic expression at the cellular level. By analyzing heterozygous knockout/reporter mice for genes with DAE, we found these effects shape genetic architecture at the cellular level in the brain and give rise to mosaics of monoallelic cells that differentially express the mutant versus wildtype alleles. Our results suggest risks for brain disorders could be influenced by the number and location of monoallelic brain cells that preferentially express mutated alleles. To determine if these effects exist in the primate brain and impact genes linked to neuropsychiatric disorders, we performed genome and transcriptome sequencing on Cynomolgus Macaque parent-offspring trios to profile putative DAEEs. We identified DAEEs for many disease linked genes and validated these effects for select genes in the human brain using allelic RNA in situ hybridization.
Our research in this area has uncovered novel non-genetic gene regulatory mechanisms that control allele-specific expression in a random manner at the cellular level. These novel effects shape genetic architecture at the cellular level in the mouse, macaque and human brain. Our ongoing research in this area is focused on learning how these novel effects influence phenotypic variation, penetrance and disease risks.
Select Papers:
a. Huang* WC, Ferris* E, Cheng T, Hörndli CS, Gleason K, Tamminga C, Wagner JD, Boucher KM, Christian JL, Gregg C. Diverse Non-genetic, Allele-Specific Expression Effects Shape Genetic Architecture at the Cellular Level in the Mammalian Brain. Neuron. 2017. 93(5):1094-1109.e7. doi: 10.1016/j.neuron.2017.01.033. PMID: 28238550 *co-authors (research article)
b. Huang WC, Bennett K and Gregg C. Epigenetic and Cellular Diversity in the Brain through Allele-Specific Effects. Trends in Neuroscience. 2018. 41(12):925-937. doi: 10.1016/j.tins.2018.07.005
Discovery of Novel Conserved Cis-Regulatory Elements and Genetic Circuits Shaping Clinical Phenotypes Using Phylogenomics.
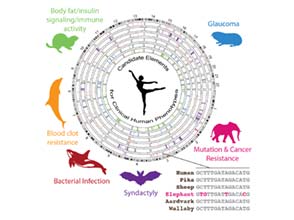
In evolution, phenotypes are predominantly shaped by cis-regulatory elements that create genetic circuits controlling the expression of networks of genes. Cis-elements have major roles in human disease, yet are poorly understood and not considered therapeutic targets. Indeed, the identities of key cis-elements and genetic circuits in mammalian genome that can control important biomedically important traits are largely unknown. My lab devised innovative methods to analyze genome-wide patterns of accelerated evolution in species that evolved “biomedical superpowers”. To date, we have identified accelerated regions (ARs) in over 15 different mammalian species. Our phylogenomics approach revealed gene regulatory mechanisms for controlling cancer risks and somatic mutations from elephants, metabolism from hibernators, intravascular blood clot resistance from deep diving dolphins, digit developmental abnormalities from bats and many other clinically important phenotypes. From this work, the Gregg lab constructed an atlas of conserved putative cis-regulatory elements for shaping different human disease phenotypes.
Our research in this area has uncovered at atlas of novel conserved cis-elements and genetic circuits for controlling disease risks. In ongoing research, we are building new high throughput screening technologies and in vivo functional assays to study the functions of the cis-elements and genetics circuits we uncovered. Major areas of focus are Alzheimer’s disease, feeding, cancer and anxiety.
Select Papers:
a. Ferris E, Abegglen L, Schiffman JD, Gregg C. Accelerated Evolution in Distinctive Species Reveals Candidate Elements for Clinically Relevant Traits, including Mutation and Cancer Resistance. Cell Reports. 2018. 22(10): 2742-2755. doi: 10.1016/j.celrep.2018.02.008, PMID: 29514101 (research article) – highlighted in international news and selected as one of the breakthroughs of year by STAT news; top accessed Cell Reports article for two months
b. Ferris E and Gregg C. Parallel Accelerated Evolution in Distant Hibernators Reveals Candidate Cis-Elements and Genetic Circuits Regulating Mammalian Obesity. Cell Reports. 2019. 29(9):2608-2620.e4. doi: 10.1016/j.celrep.2019.10.102, PMID: 31775032 (research article) – selected for the cover of the journal; top accessed Cell Reports article for one month
Development of Novel Assays and Machine learning Approaches for Behavioral Genetics and Epigenetics to Learn How Complex Ethological Behavior Patterns are Constructed

Most studies of behavior focus on analyzing specific behavioral responses and as a consequence the principles and mechanisms involved in shaping complex ethological patterns are poorly defined. My lab developed a naturalistic foraging behavior assay that challenges a wide range of neural systems, including feeding, exploration, fear, activity, perseveration, learning & memory and sensorimotor abilities. Mice are video recorded in the assay. We developed a machine learning and data analysis pipeline to study complex behavior patterns called DeepFeats, which integrates hundreds of features of the behavior, including movement patterns and environmental context information, and then segments the behavior into finite behavioral sequences based on ethological landmarks, like the home. DeepFeats then performs statistical tests to discover significantly reproducible behavioral sequences that we call “modules”. Our work reveals that complex behavior patterns are constructed from finite modules that range from less than a second in duration to hundreds of seconds in duration. Modules are expressed in a probabilistic determined sequential order, revealing rules for pattern construction. We found that genetic and epigenetic mechanisms can be mapped on specific modules.
Our research in this area has established the foundations for a radial new approach to behavioral genetics and epigenetics that is expected to reveal how genes and cis-elements shape complex behavior patterns. Our ongoing work is focused on improving DeepFeats for behavioral genetics applications and studying how different genetic and epigenetic mechanisms control module form, expression frequency, order and timing.
Select Papers:
a. Cornelia N Stacher Hörndli, Eleanor Wong, Elliott Ferris, Kathleen Bennett, Susan Steinwand, Alexis Nikole Rhodes, P. Thomas Fletcher and Christopher Gregg. Complex Economic Behavior Patterns are Constructed From Finite, Genetically Controlled Modules of Behavior. Cell Reports. 2019. 13;28(7):1814-1829.e6. doi: 10.1016/j.celrep.2019.07.038. PMID 31412249 (research article) – selected for the cover of the journal
b. Gregg C. Machine Learning Within Behavioral Sequencing. United States Patent and Trademark Office Serial No. 62/873,098
LAB APPLICATION